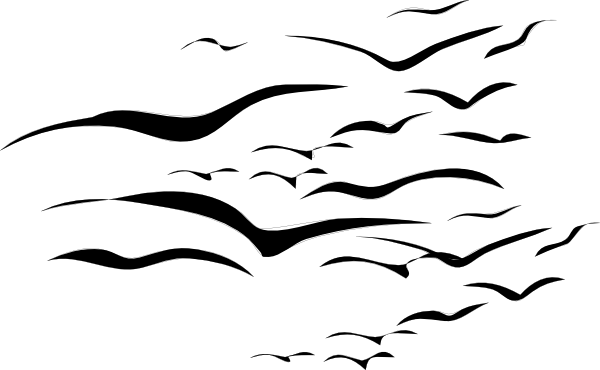
Swarms in Nature
Swarm Intelligence has its roots in the natural world: that is where the first swarms were, after all. Swarming bees, shoals of fish and flocking birds all use swarms in interesting ways, but we shall start by taking a look at what ants gain from living collectively. Pay close attention to the natural mechanisms described, because in Chapter 4 they will be adapted and built on for swarm-based technology.
One remarkable thing about ants is that although no single ant is in charge, they always send the right number of foragers out of the nest. The number will vary depending on the conditions on a certain day. For example if there isn’t much food to be collected then fewer ants will be required, but if the nest has been damaged then more ants are needed to go out and fix it. The question as to how they do it is tricky, but the answer turns out to be quite simple. Deborah Gordon has been researching red harvester ants (Pogonomyrmex barbatus) in the Arizona desert. Every morning a class of patroller ants leaves the nest. These patrollers have a distinctive hydrocarbon scent coating their bodies, and their job is to scout the area surrounding the nest for food. As soon as they find some food, they return to the nest. Thus the more food there is scattered around, the more frequently the patrollers will return to the nest. Forager ants waiting in the nest entrance are somehow provoked to leave the nest by the returning patrollers. Gordon wanted to know exactly how the foragers were stimulated to leave the nest, so one morning she captured the patrollers after they left the nest and performed an experiment. She dropped beads into the nest entrance. The first thing she found was that the foragers only left the nest as a response to contact with beads coated in the patroller scent; there was no reaction to the foragers being jostled by scentless beads, showing that they recognise the patroller scent. Next she varied the rate at which the beads were dropped. She found that if the time interval between dropping each bead was either too long or too short the foragers would not leave. The optimum rate was one bead every ten seconds, which resulted in the foragers gushing out from the nest. The explanation is this: drop the beads in too slowly (corresponding to a scarcity of food) and the foragers will stay in the nest because by the time a second bead touches an ant it will already have forgotten the first. But the forager ants are programmed to sit tight if they are jostled too much (when the beads are dropped in too fast). This is beneficial because the usual cause of the over-contact would be the patrollers rushing back into the nest because of a danger outside, such as a predator. So the ants use a decentralised method based on local interactions between patrollers and foragers to fine tune the number of foragers leaving the nest, proving that it’s possible to coordinate complex actions without having someone in charge. [1] [5]
Ants have the uncanny ability to select the shortest route from a food source to their nest. This is exhibited in the ant ‘highways’ seen often in the wild and sometimes in our kitchens. This is another example of how large numbers of simple agents with limited intelligence can perform remarkable feats whereas humans often struggle to find the shortest route. Take the Argentine ant (Linepithema humile), investigated by Jean-Louis Deneubourg of the Free University of Brussels. When this ant forages it deposits a trail of chemicals called pheromones. The ant will lay the pheromone trail on the way out to find food and then return along the same trail, meaning that when they return to the nest there will be a double-strength layer of pheromone. Other ants foraging for food will smell the pheromone trail and follow it, they themselves adding to the strength of the smell. But this situation is not yet quite adequate, because as it stands all the ants would follow one trail which would be continually reinforced in a positive feedback loop. There’s a trick to stopping this loop, allowing the ants to find a short route: evaporation. The pheromone strength decreases over time. This means that if one ant takes a long route and one a short route to the same piece of food, then at the time when each ant gets back to the nest the pheromone trail marking the longer route will be weaker. This is because the ant on the long route took more time, allowing more of the pheromone to evaporate. Thus the next forager ants to leave the nest will be more likely to select the stronger smelling, shorter route. And so where humans would need to spend time pouring over a map (and making the map in the first place), ants succeed with some style - just by having a set of simple rules which govern local interactions. [6] This method holds a likeness to humans following a well-worn path in the woods, but a distinction does exist. The first human to pick a path used their intellect to try to pick a good route, and their footfalls began to erode the ground into a path. The first ant, however, acts more blindly; the ants rely on their large numbers working in concert to allow a quick route to emerge. With a lack of intellect they throw their resources at the problem, and it’s an effective strategy. (This idea is elaborated in Chapter 3, concerning the random quality in swarms.)
Don’t be too envious; sometimes these simple rules are the downfall of an ant. For example - what would happen if an ant took a few chance turns and ended up following its own pheromone trail? The sad news is that the ant ends up going round and round in an endless circle, continually adding to the strength of the trail, and enticing other ants in. [5] These so-called ant ‘mills’ can spell death to large chunks of a colony, and they have echoes in computing - programs can get stuck in ‘infinite loops’, possibly causing your computer to crash.
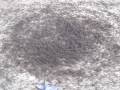
Perhaps the most miraculous examples of Swarm Intelligence in nature are the honeybees (Apis mellifera) - in particular, when they decide to move house. This normally happens during late springtime: the colony, grown in size, gets too crowded and splits, leaving half the bees homeless. The dislodged bees settle temporarily in a sheltered location, while scouts go out to search for a new nest site. The scouts are looking for somewhere high up with a small entrance hole (a tree cavity for instance) that has enough room inside. Thomas Seeley, a biologist at Cornell University, led a team on an experiment to find out what happens next. Seeley brought several honeybee swarms to Appledore Island, off the coast of Maine in the States. The island has very few trees and no natural nest sites for the bees.

The team had marked every bee with a paint dot and a small plastic identification tag, and so they began by setting up five artificial nesting sites for the bees to choose from. Four were designed to be the wrong size, but the fifth was made just right. They released the bee swarm, and through careful observation discovered how the bees move house. Each scout scours the land until it discovers a possible nest. The bee examines the site briefly, and if it determines that the site is suitable it will return to the swarm, where the strange part happens. The scout bees perform a routine - a ‘waggle dance’ - to convey that they found a nest site to other scouts. The dance also contains a code, containing the directions to the site. The waggle dance is in a figure of eight shape and, somewhat unbelievably, the angle that the straight mid-section of the eight makes with the top of the nest tells the other bees what angle their flight should make with the sun. The length of the mid-section indicates the distance.
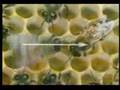
The scouts that watched the dance then go to check out the advertised nest site, and if they agree that it’s a good one then they return to the swarm to dance for it themselves. Through Seeley’s experiment and a number of similar ones, it turns out that there is a critical mass. As soon as about 15 scouts converge on one of the nest sites simultaneously, they sense that an agreement has been made, and they return to the swarm to bring them to the new nest site. Unsurprisingly, in Seeley’s experiment the best sized site was settled on.
The honeybees use a distributed method to make their important nesting decision. This confers a lot of advantages. If there was one bee in charge of choosing the new site, it would need to scour the entire island to find the best site, and if it got lost the rest of the swarm would be out of luck. Using the bees’ method the task of searching the island is split up, and no single bee has an overview of the whole process. This means when looking for a solution to the problem, the process is resilient to failure as a few dead bees will not affect any of the local interactions on a wider scale. This is one of the attractive sides to using swarms in software and robot design, but that will come later. Crucially, the decision-making process is an approximating one - the best nest site may not be found, but the bees will tend to find very good ones very quickly. [1] [7]
Another point of interest in the case of the bees is language. In Karl Popper’s essay Of Clouds and Clocks, he discusses how language can be divided into lower and higher functions. There are two lower functions, within the reach of many animals. The first is the ability to convey a symptom by making some form of signal; a wolf in pain may squeal, for instance. The second is the ability to respond to such a signal; other wolves may come to the rescue. The two higher functions are more elaborate: description and argument. The lower functions are always present in communication, but it is usually only humans who are able to describe something or argue about what’s right. [8] But now let’s look at the bees. Whenever a bee performs the waggle dance, it is actually describing the whereabouts of the nest site. Impressive, but this individual bee is not capable of the other higher function - argument. Nevertheless the bees do reach a decision about the new nest site: while each bee is not capable of arguing, collectively the swarm is. This is a great illustration of how simple agents can collaborate (or swarm) to overcome limitations on their intelligence, and it speaks volumes for the potential of collaborative design, say, in robotics.
A superorganism is “a collection of agents [which] could act in concert to produce phenomena governed by the collective.” [9] Fish in a shoal form a superorganism very well adapted to avoiding predators. The more pairs of eyes in the shoal, the more alert it is to danger. When a fish sees a shark it will dart out the way; the neighbouring fish also dart out the way in response to the first fish. This domino effect causes shockwaves to ripple through the shoal, and every fish is made aware of danger staggeringly quickly. The shoal uses its swarm nature to its advantage by behaving as a superorganism. Many behave as one, and the shoal has tricks that make it extremely difficult for a predator to track any one fish. For example, it may form a bubble around the predator, or explode, sending fish in all directions. [5]
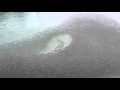
These tricks, and the superorganism itself, are all examples of the complex behaviour which emerges from a swarm. They result from the local interactions between the agents, the fish responding to their neighbours.
Birds also display such emergent behaviour, like when starlings wheel across the sky in huge flocks.
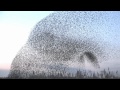
There are several reasons starlings perform these aerial acrobatics: to intimidate and bamboozle predators, to attract more starlings for greater safety in numbers, and perhaps as a form of socialising - starlings are more intelligent than ants after all. [10] But in 1986 a computer graphics researcher, Craig Reynold, was more interested in how the starlings flocked together than why, and he wanted to make a realistic computer simulation. He knew the behaviour had to emerge from each bird following simple rules, because each bird only has access to local information about other birds in its immediate vicinity. So with this in mind he sought to construct a model where each agent follows a limited number of rules. The agents in this model are called boids (birds in a New York accent), and they live in a three dimensional world. Reynolds whittled down to only three rules necessary for each boid: first, steer away from nearby boids if you are much too close (to avoid getting in their way); second, steer towards nearby boids so long as you are not too close already; and third, steer so as to head in the same general direction as nearby boids. It turned out that a good balance of these simple rules yielded a remarkably convincing model of a flock of birds. Unpredictable, life-like movements had emerged. Observation and experiment with real starlings has confirmed that these rules are the ones they follow. [5] [11] Boids’ feature film debut came as a swarm of computer-generated bats in the 1992 film ‘Batman Returns’; it is still used in films and video games.
I have developed my own rendition of Reynolds’ Boids, written entirely in Javascript. The app is based on the same principles: nearby agents try to stay close, but not too close, and they try to move in the same direction. I call these attraction, repulsion, and direction matching. But there are four key differences. First, my version is two dimensional, but still serves as a good representation of emergence and flocking behaviour. Second, my version is built on top of a physics engine, which is what brings real-world physics (for instance gravity) to computer simulations. What this means for my app is that the agents can collide with each other and bounce off. The third difference is that I have implemented the three rules of attraction, repulsion and direction matching in a different way: Reynolds uses the rules to affect the steering of each boid, but my app directly changes the velocities. To explain this better: imagine the boids are cars. For a boid to change direction completely in Reynolds’ simulation, it must perform a U-turn. In my app the agents can stop and put the car in reverse gear, thus moving off backwards without leaving the same straight line. This is why Reynolds’ boids look more like flocking birds, whereas my agents more closely resemble swarming insects (which are more manoeuvrable). The fourth difference is that my app is interactive. We do not suffer from the hardware constraints that Reynolds did in the 1980s, so I was able to add parameters which can be tinkered with to alter the animation in real-time. For example you can change the strength of attraction, the radius of repulsion (how close other agents must be before they will be repelled), and the number of agents. Furthermore, Reynolds boids had a fixed field of view (that is, they could not see behind them), whereas I have made it adjustable. 360° corresponds to being able to see in every direction, and 0° makes the boids blind. Reynolds had to deliver the finished product: you click go and you’ve got a flock of birds. My app on the other hand allows the user to tweak the controls in order to build their own flock, so the user garners an appreciation of how finely-tuned the rules of local interaction must be for emergent behaviour to develop. With care the agents can be made to form a convincing bird flock.
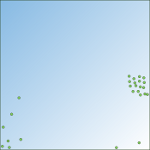
If you were sceptical of the principle of emergence, the app should change your mind. It is a manifestation of complex behaviour emerging from simple rules - and in this case, we made the rules! [Edit 15.10.11: I've updated the app to include the option of a predator.]
One remarkable thing about ants is that although no single ant is in charge, they always send the right number of foragers out of the nest. The number will vary depending on the conditions on a certain day. For example if there isn’t much food to be collected then fewer ants will be required, but if the nest has been damaged then more ants are needed to go out and fix it. The question as to how they do it is tricky, but the answer turns out to be quite simple. Deborah Gordon has been researching red harvester ants (Pogonomyrmex barbatus) in the Arizona desert. Every morning a class of patroller ants leaves the nest. These patrollers have a distinctive hydrocarbon scent coating their bodies, and their job is to scout the area surrounding the nest for food. As soon as they find some food, they return to the nest. Thus the more food there is scattered around, the more frequently the patrollers will return to the nest. Forager ants waiting in the nest entrance are somehow provoked to leave the nest by the returning patrollers. Gordon wanted to know exactly how the foragers were stimulated to leave the nest, so one morning she captured the patrollers after they left the nest and performed an experiment. She dropped beads into the nest entrance. The first thing she found was that the foragers only left the nest as a response to contact with beads coated in the patroller scent; there was no reaction to the foragers being jostled by scentless beads, showing that they recognise the patroller scent. Next she varied the rate at which the beads were dropped. She found that if the time interval between dropping each bead was either too long or too short the foragers would not leave. The optimum rate was one bead every ten seconds, which resulted in the foragers gushing out from the nest. The explanation is this: drop the beads in too slowly (corresponding to a scarcity of food) and the foragers will stay in the nest because by the time a second bead touches an ant it will already have forgotten the first. But the forager ants are programmed to sit tight if they are jostled too much (when the beads are dropped in too fast). This is beneficial because the usual cause of the over-contact would be the patrollers rushing back into the nest because of a danger outside, such as a predator. So the ants use a decentralised method based on local interactions between patrollers and foragers to fine tune the number of foragers leaving the nest, proving that it’s possible to coordinate complex actions without having someone in charge. [1] [5]
Ants have the uncanny ability to select the shortest route from a food source to their nest. This is exhibited in the ant ‘highways’ seen often in the wild and sometimes in our kitchens. This is another example of how large numbers of simple agents with limited intelligence can perform remarkable feats whereas humans often struggle to find the shortest route. Take the Argentine ant (Linepithema humile), investigated by Jean-Louis Deneubourg of the Free University of Brussels. When this ant forages it deposits a trail of chemicals called pheromones. The ant will lay the pheromone trail on the way out to find food and then return along the same trail, meaning that when they return to the nest there will be a double-strength layer of pheromone. Other ants foraging for food will smell the pheromone trail and follow it, they themselves adding to the strength of the smell. But this situation is not yet quite adequate, because as it stands all the ants would follow one trail which would be continually reinforced in a positive feedback loop. There’s a trick to stopping this loop, allowing the ants to find a short route: evaporation. The pheromone strength decreases over time. This means that if one ant takes a long route and one a short route to the same piece of food, then at the time when each ant gets back to the nest the pheromone trail marking the longer route will be weaker. This is because the ant on the long route took more time, allowing more of the pheromone to evaporate. Thus the next forager ants to leave the nest will be more likely to select the stronger smelling, shorter route. And so where humans would need to spend time pouring over a map (and making the map in the first place), ants succeed with some style - just by having a set of simple rules which govern local interactions. [6] This method holds a likeness to humans following a well-worn path in the woods, but a distinction does exist. The first human to pick a path used their intellect to try to pick a good route, and their footfalls began to erode the ground into a path. The first ant, however, acts more blindly; the ants rely on their large numbers working in concert to allow a quick route to emerge. With a lack of intellect they throw their resources at the problem, and it’s an effective strategy. (This idea is elaborated in Chapter 3, concerning the random quality in swarms.)
Don’t be too envious; sometimes these simple rules are the downfall of an ant. For example - what would happen if an ant took a few chance turns and ended up following its own pheromone trail? The sad news is that the ant ends up going round and round in an endless circle, continually adding to the strength of the trail, and enticing other ants in. [5] These so-called ant ‘mills’ can spell death to large chunks of a colony, and they have echoes in computing - programs can get stuck in ‘infinite loops’, possibly causing your computer to crash.
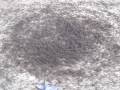
Perhaps the most miraculous examples of Swarm Intelligence in nature are the honeybees (Apis mellifera) - in particular, when they decide to move house. This normally happens during late springtime: the colony, grown in size, gets too crowded and splits, leaving half the bees homeless. The dislodged bees settle temporarily in a sheltered location, while scouts go out to search for a new nest site. The scouts are looking for somewhere high up with a small entrance hole (a tree cavity for instance) that has enough room inside. Thomas Seeley, a biologist at Cornell University, led a team on an experiment to find out what happens next. Seeley brought several honeybee swarms to Appledore Island, off the coast of Maine in the States. The island has very few trees and no natural nest sites for the bees.

The team had marked every bee with a paint dot and a small plastic identification tag, and so they began by setting up five artificial nesting sites for the bees to choose from. Four were designed to be the wrong size, but the fifth was made just right. They released the bee swarm, and through careful observation discovered how the bees move house. Each scout scours the land until it discovers a possible nest. The bee examines the site briefly, and if it determines that the site is suitable it will return to the swarm, where the strange part happens. The scout bees perform a routine - a ‘waggle dance’ - to convey that they found a nest site to other scouts. The dance also contains a code, containing the directions to the site. The waggle dance is in a figure of eight shape and, somewhat unbelievably, the angle that the straight mid-section of the eight makes with the top of the nest tells the other bees what angle their flight should make with the sun. The length of the mid-section indicates the distance.
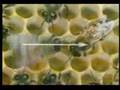
The scouts that watched the dance then go to check out the advertised nest site, and if they agree that it’s a good one then they return to the swarm to dance for it themselves. Through Seeley’s experiment and a number of similar ones, it turns out that there is a critical mass. As soon as about 15 scouts converge on one of the nest sites simultaneously, they sense that an agreement has been made, and they return to the swarm to bring them to the new nest site. Unsurprisingly, in Seeley’s experiment the best sized site was settled on.
The honeybees use a distributed method to make their important nesting decision. This confers a lot of advantages. If there was one bee in charge of choosing the new site, it would need to scour the entire island to find the best site, and if it got lost the rest of the swarm would be out of luck. Using the bees’ method the task of searching the island is split up, and no single bee has an overview of the whole process. This means when looking for a solution to the problem, the process is resilient to failure as a few dead bees will not affect any of the local interactions on a wider scale. This is one of the attractive sides to using swarms in software and robot design, but that will come later. Crucially, the decision-making process is an approximating one - the best nest site may not be found, but the bees will tend to find very good ones very quickly. [1] [7]
Another point of interest in the case of the bees is language. In Karl Popper’s essay Of Clouds and Clocks, he discusses how language can be divided into lower and higher functions. There are two lower functions, within the reach of many animals. The first is the ability to convey a symptom by making some form of signal; a wolf in pain may squeal, for instance. The second is the ability to respond to such a signal; other wolves may come to the rescue. The two higher functions are more elaborate: description and argument. The lower functions are always present in communication, but it is usually only humans who are able to describe something or argue about what’s right. [8] But now let’s look at the bees. Whenever a bee performs the waggle dance, it is actually describing the whereabouts of the nest site. Impressive, but this individual bee is not capable of the other higher function - argument. Nevertheless the bees do reach a decision about the new nest site: while each bee is not capable of arguing, collectively the swarm is. This is a great illustration of how simple agents can collaborate (or swarm) to overcome limitations on their intelligence, and it speaks volumes for the potential of collaborative design, say, in robotics.
A superorganism is “a collection of agents [which] could act in concert to produce phenomena governed by the collective.” [9] Fish in a shoal form a superorganism very well adapted to avoiding predators. The more pairs of eyes in the shoal, the more alert it is to danger. When a fish sees a shark it will dart out the way; the neighbouring fish also dart out the way in response to the first fish. This domino effect causes shockwaves to ripple through the shoal, and every fish is made aware of danger staggeringly quickly. The shoal uses its swarm nature to its advantage by behaving as a superorganism. Many behave as one, and the shoal has tricks that make it extremely difficult for a predator to track any one fish. For example, it may form a bubble around the predator, or explode, sending fish in all directions. [5]
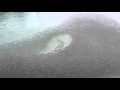
These tricks, and the superorganism itself, are all examples of the complex behaviour which emerges from a swarm. They result from the local interactions between the agents, the fish responding to their neighbours.
Birds also display such emergent behaviour, like when starlings wheel across the sky in huge flocks.
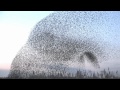
There are several reasons starlings perform these aerial acrobatics: to intimidate and bamboozle predators, to attract more starlings for greater safety in numbers, and perhaps as a form of socialising - starlings are more intelligent than ants after all. [10] But in 1986 a computer graphics researcher, Craig Reynold, was more interested in how the starlings flocked together than why, and he wanted to make a realistic computer simulation. He knew the behaviour had to emerge from each bird following simple rules, because each bird only has access to local information about other birds in its immediate vicinity. So with this in mind he sought to construct a model where each agent follows a limited number of rules. The agents in this model are called boids (birds in a New York accent), and they live in a three dimensional world. Reynolds whittled down to only three rules necessary for each boid: first, steer away from nearby boids if you are much too close (to avoid getting in their way); second, steer towards nearby boids so long as you are not too close already; and third, steer so as to head in the same general direction as nearby boids. It turned out that a good balance of these simple rules yielded a remarkably convincing model of a flock of birds. Unpredictable, life-like movements had emerged. Observation and experiment with real starlings has confirmed that these rules are the ones they follow. [5] [11] Boids’ feature film debut came as a swarm of computer-generated bats in the 1992 film ‘Batman Returns’; it is still used in films and video games.
I have developed my own rendition of Reynolds’ Boids, written entirely in Javascript. The app is based on the same principles: nearby agents try to stay close, but not too close, and they try to move in the same direction. I call these attraction, repulsion, and direction matching. But there are four key differences. First, my version is two dimensional, but still serves as a good representation of emergence and flocking behaviour. Second, my version is built on top of a physics engine, which is what brings real-world physics (for instance gravity) to computer simulations. What this means for my app is that the agents can collide with each other and bounce off. The third difference is that I have implemented the three rules of attraction, repulsion and direction matching in a different way: Reynolds uses the rules to affect the steering of each boid, but my app directly changes the velocities. To explain this better: imagine the boids are cars. For a boid to change direction completely in Reynolds’ simulation, it must perform a U-turn. In my app the agents can stop and put the car in reverse gear, thus moving off backwards without leaving the same straight line. This is why Reynolds’ boids look more like flocking birds, whereas my agents more closely resemble swarming insects (which are more manoeuvrable). The fourth difference is that my app is interactive. We do not suffer from the hardware constraints that Reynolds did in the 1980s, so I was able to add parameters which can be tinkered with to alter the animation in real-time. For example you can change the strength of attraction, the radius of repulsion (how close other agents must be before they will be repelled), and the number of agents. Furthermore, Reynolds boids had a fixed field of view (that is, they could not see behind them), whereas I have made it adjustable. 360° corresponds to being able to see in every direction, and 0° makes the boids blind. Reynolds had to deliver the finished product: you click go and you’ve got a flock of birds. My app on the other hand allows the user to tweak the controls in order to build their own flock, so the user garners an appreciation of how finely-tuned the rules of local interaction must be for emergent behaviour to develop. With care the agents can be made to form a convincing bird flock.
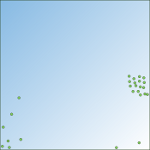
If you were sceptical of the principle of emergence, the app should change your mind. It is a manifestation of complex behaviour emerging from simple rules - and in this case, we made the rules! [Edit 15.10.11: I've updated the app to include the option of a predator.]